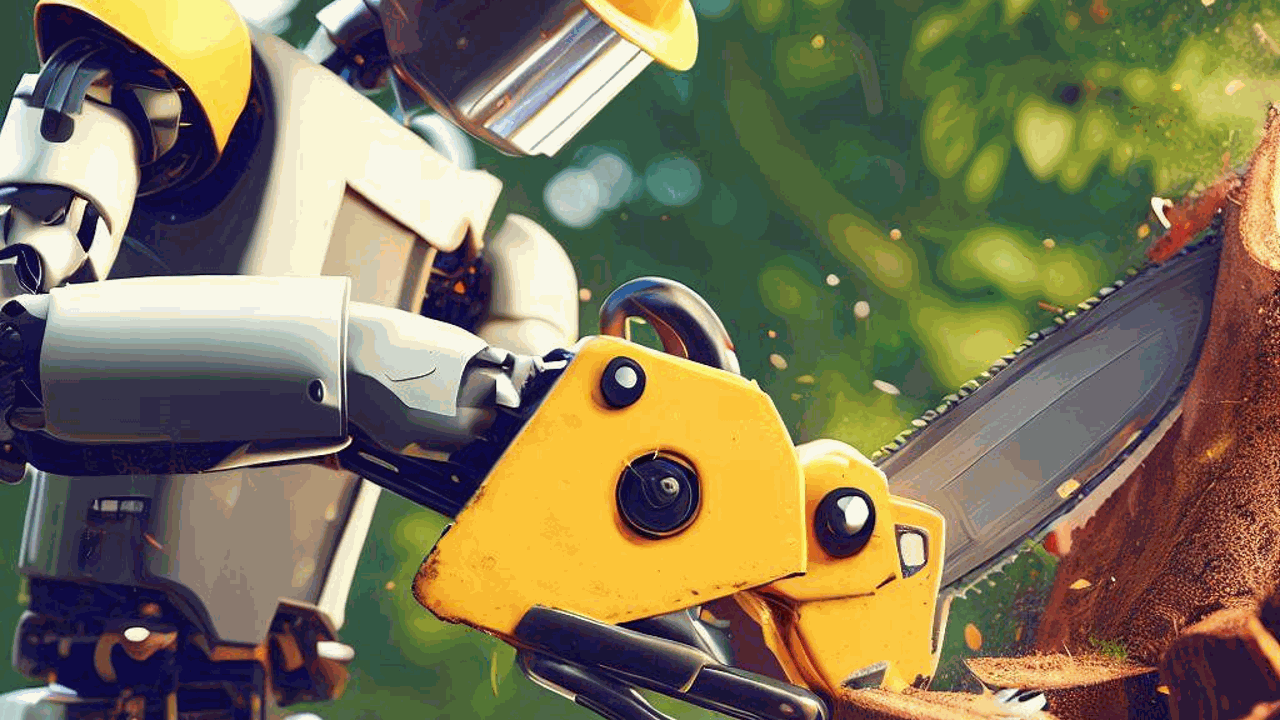
Does Random Forest Need Feature Scaling or Normalization?
If you are using Random Forest as your machine learning model, you don’t need to worry about scaling or normalizing your features. Random Forest is a tree-based model and hence does not require feature scaling. Tree-based models are invariant to the scale of the features, which makes them very user-friendly as this step can be skipped during preprocessing. Still, in practice you can see different results when you scale your features because of the way numerical values are represented in computers....